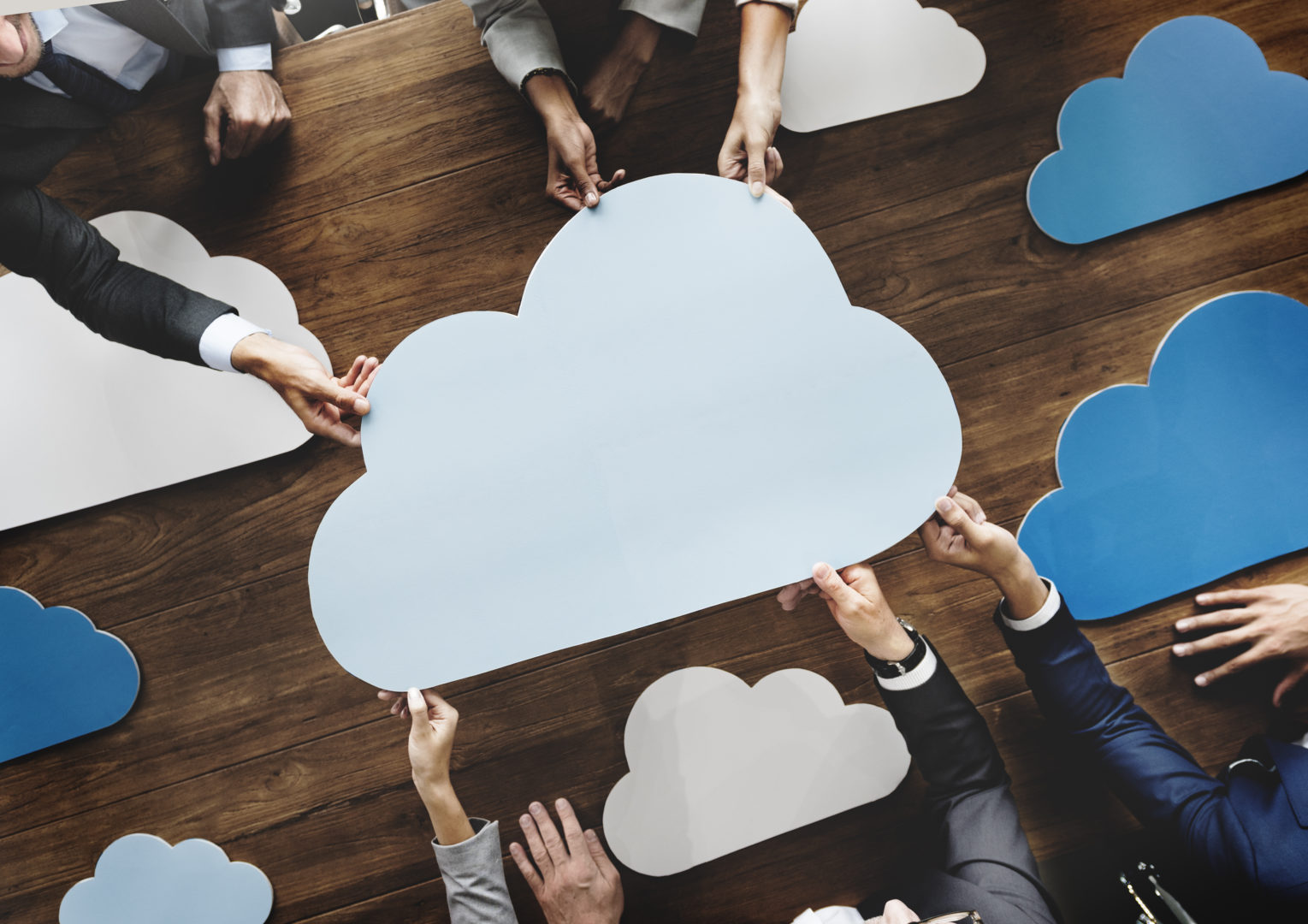
The De-mythification Series Part 4: The Automagic Pixie
Au-to-mag-ic: (Of a usually complicated technical or computer process) done, operating, or happening in a way that is hidden from or not understood by the user, and in that sense, apparently magical
[Source: Dictionary.com]
In previous installments of this series, I de-bunked some of the more common myths around big data analytics. In this final installment, I’ll address one of the most pervasive and costly myths: that there exists an easy button that organizations can press to automagically solve their big data problems. I’ll provide some insights as to how this myth has come about, and recommend strategies for dealing with the real challenges inherent in big data analytics.
Like the single-solution elf, this easy button idea is born of the desire of many vendors to simplify their message. The big data marketplace is new enough that all the distinct types of needs havent yet become entirely clear—which makes it tough to formulate a targeted message. Remember in the late 1990s when various web vendors were all selling “e-commerce” or ”narrowcasting” or “recontextualization”? Today most people are clear on the utility of the first two, while the third is recognized for what it was at the time—unhelpful marketing fluff. I worked with a few of these firms, and watched as the businesses tried to position product for a need which hadn’t yet been very well defined by the marketplace. The typical response by the business was to keep it simple—just push the easy button and our technology will do it for you.
I was at my second startup in 2001 (an e-commerce provider using what we would refer to today as a SaaS model) when I encountered the unfortunate aftermath of this approach. I sat down at my desk on the first day of the job, and was promptly approached by the VP of Engineering, who informed me that our largest customer was about to cancel its contract—we’d been trying to upgrade the customer for weeks, during which time their e-commerce system was down. Although they’d informed the customer that the upgrade was a push-button process, it wasn’t. In fact, at the time I started there, the team was starting to believe that an upgrade would be impossible and that they should propose re-implementing the customer from scratch. By any standard, this would be a fail.
Over the next 72 hours, I migrated the customer’s data and got them up and running. It was a Pyrrhic victory at best—the customer cancelled anyhow, and the startup went out of business a few months later.
The moral of the story? No, it’s not to keep serious data geeks on staff to do automagical migrations. The lesson here is that when it comes to data driven applications—including analytics—the too good to be true easy button almost always is. Today, the big data marketplace is full of great sounding messages such as ”up and running in minutes”, or ”data scientist in a box.”
“Push a button and deploy a big data infrastructure in minutes to grind through that ten petabytes of data sitting on your SAN!”
“Automatically derive predictive models that used to take the data science team weeks in mere seconds! (…and then fire the expensive data scientists)!”
Don’t these sound great?
The truth is, as usual, more nuanced. One key point I like to make with organizations is that big data analytics, like most technology practices, involves different tasks. And those tasks generally require different tools. To illustrate this for business stakeholders, I usually resort to the metaphor of building a house. We don’t build a house with just a hammer, or just a screwdriver. In fact, it requires a variety of tools—each of which is suited to a different task. A brad nailer for drywall. A circular saw for cutting. A framing hammer for framing. And so on. And in the world of engineering, a house is a relatively simple thing to construct. A big data infrastructure is considerably more complex. So it’s reasonable to assume that an organization building this infrastructure would need a rich set of tools and technologies to meet the different needs.
Now that we’ve clarified this, we can get to the question behind the question. When someone asks me ”Why can’t we have an easy button to build and deploy analytics?” What they’re really asking is “How can I use technological advances to build and deploy analytics faster, better and cheaper?”
Ahh, now that’s an actionable question!
In the information technology industry, we’ve been blessed (some would argue cursed) by the nature of computing. For decades now we’ve been able to count on continually increasing capacity and efficiency. So while processors continue to grow more powerful, they also consume less power. As the power requirements for a given unit of processing become low enough, it is suddenly possible to design computing devices which run on “ambient” energy from light, heat, motion, etc. This has opened up a very broad set of possibilities to instrument the world in ways never before seen—resulting in dramatic growth of machine-readable data. This data explosion has led to continued opportunity and innovation across the big data marketplace. Imagine if each year, a homebuilder could purchase a saw which could cut twice as much wood with a battery half the size. What would that mean for the homebuilder? How about the vendor of the saw? Thats roughly analogous to what we all face in big data.
And while we won’t find one ”easy button,” its very likely that we can find a tool for a given analytic task which is significantly better than one that was built in the past. A database that operates well at petabyte scale, with performance characteristics that make it practical to use. A distributed filesystem whose economics make it a useful place to store virtually unlimited amounts of data until you need it. An engine capable of extracting machine-readable structured information from media. And so on. Once my colleagues and I have debunked the myth of the automagic pixie, we can have a productive conversation to identify the tools and technologies that map cleanly to the needs of an organization and can offer meaningful improvements in their analytical capability.
I hope readers have found this series useful. In my years in this space, I’ve learned that in order to move forward with effective technology selection, sometimes we have to begin by taking a step backward and undoing misconceptions. And there are plenty! So stay tuned.