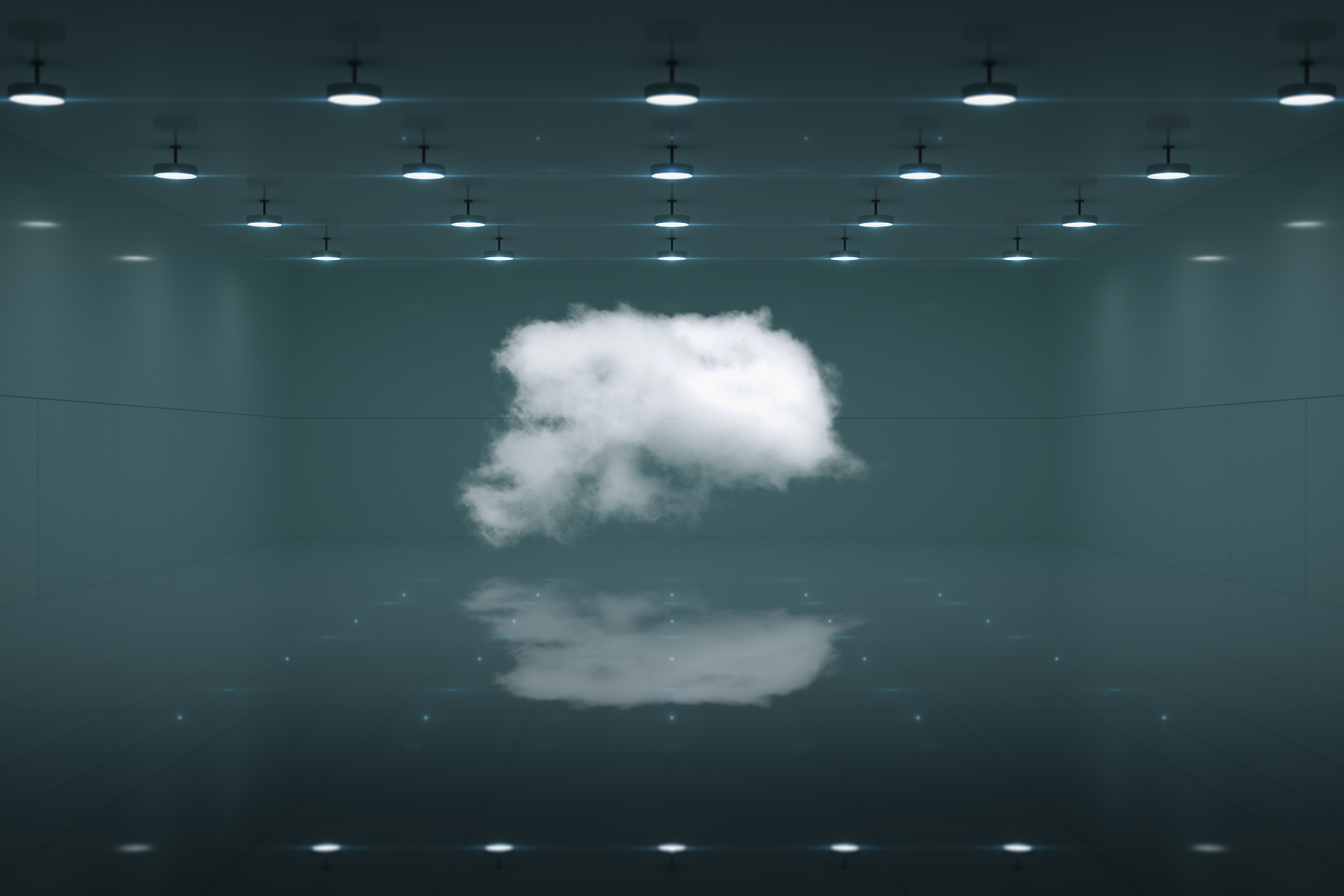
Is Big Data Giving You Grief? Part 3: Bargaining
“Can’t we work with our current technologies (and vendors)? But they cost too much!”
Continuing the five part series about the stages of big-data grief that organizations experience, this segment focuses on the first time organizations explore the reality of the challenges and opportunities presented by big data and start to work their way forward .. with bargaining.
Coping with a missed opportunity often brings some introspection. And with that comes the need to explore what-ifs that may provide a way forward. Here are some of the more common what-ifs that organizations explore during this phase.
What if I go to my current vendor? Theyre sure to have some great technology. That’ll fix the problem.
This is a perfectly fine path of inquiry to explore. The only issue with this, as mentioned in my previous post (Part 2: Anger) is that vendors have a tendency to re-label their technology to suit a desirable market. So their technology offerings may not actually be suited to big data needs. And spending time and effort exploring these technologies to verify this can distract and prevent you from moving forward.
Also, vendors may have a business that relies on high-margin technology or services that were priced for a time before the big data explosion. So, the economics of their technology may suit them, but not the organization in need—your company. For example, if I need to store a petabyte of data in a data warehouse, I might require a several hundred node data warehouse cluster. If my current vendor charges a price of a hundred thousand US dollars per node, this isn’t economically feasible since I can now find alternatives that are purpose-built for large scale database processing and are priced at 1/5th or 1/10th that (or less!).
What if I hire some smart people? They’ll bring skills and insight. They’ll fix the problem.
Like the question above, this is a perfectly reasonable question to ask. But hiring bright people with the perfect skills can be very difficult today—the talent pool for big data is slim, and the hiring for these folks is highly competitive. Furthermore, hiring from outside doesn’t bring in the context of the business. In almost every business, there are nuances to the products, culture, market, and so forth that have a meaningful impact on the business. Hired guns, no matter how skilled, often lack this context.
Also, just bringing in new people doesn’t necessarily mean that your organizations technology will suit them. Most analytic professionals develop their way of operating—their “game plan”—early in their career, and often prefer a particular set of technologies. It’s likely your new hires will want to introduce technologies they’re familiar with to your organization. And that can introduce additional complexity. A classic example of this is hiring a data science team who have spent the last decade analyzing data with the SAS system. If the organization doesn’t use SAS to begin with, the new team will likely press to introduce it… And that may conflict with how the how the organization approaches analytics.
What if I download this cool open source software? I hear that stuff is magic, so that’ll fix the problem.
Unlike the first two what-ifs, this one should be approached with great caution! As mentioned in my previous post, open source software has something of a unique tendency to be associated with vague, broad, exaggerated, and often contradictory claims of functionality. This brings to mind a classic bit of satire by the Saturday Night Live crew, first aired in 1976: “New Shimmer is both a floor wax and a dessert topping!” The easy mistake to make here is for the technology team to rush forward, install the new stuff and start to experiment with it to the exclusion of all else. Six months (and several million dollars of staff time) later, the sunk cost in the open source option is so huge that it becomes a fait accompli. Careers would be damaged if the team admitted that it just wasted six months proving that the technology does not do what it claims, so it becomes the default choice.
What if I do what everybody else is doing? Crowds have wisdom, so that’ll fix the problem.
The risk with this thinking is similar to that posed by open source. This often goes hand-in-hand with hiring big data smarts—companies often bring in people from the outside and pay them to do what they’ve done elsewhere. It can definitely accelerate a big data program. But it can also guarantee that the efforts are more of a me-too duplication of something the rest of the industry has already done rather than true innovation. And while this may be suited for some businesses, the big money in big data is in being the first to derive new insights.
These are all perfectly acceptable questions that come up as organizations begin to acknowledge, for the first time, the reality of big data. But this isn’t the end of the discussion by any means. It’s important to avoid getting so enamored with exploring one or two of the above options that you don’t follow through on the “grief” process. But the natural next step is to be intimidated by the challenge, which will serve as an important reality check. Ill cover this in the next segment: depression. So stay tuned!
Next up: Depression ”The problem is too big. How can we possibly tackle it?”