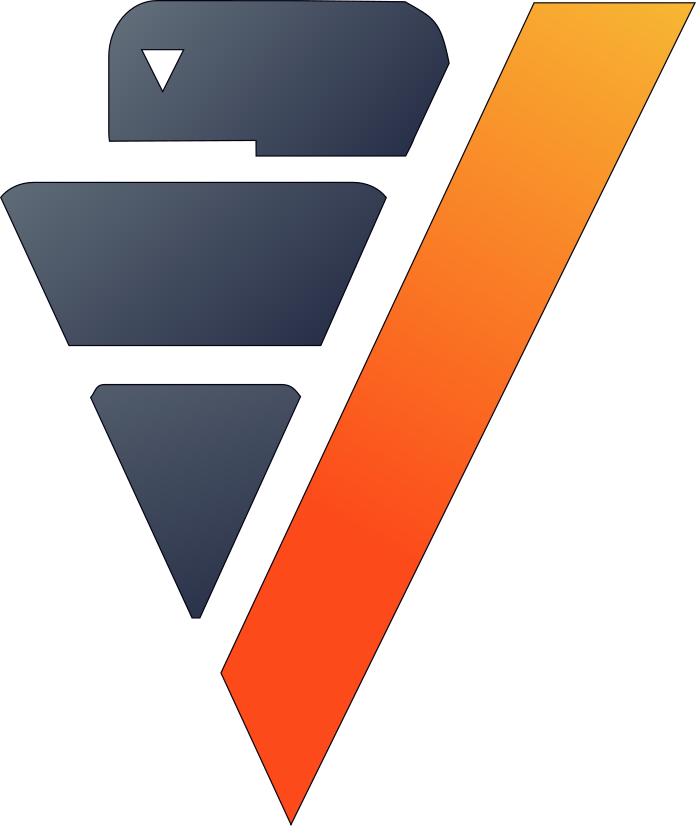
VerticaPy
Quick Start¶
First, install the API using the pip command.
root@ubuntu:~$ pip3 install verticapy[all]
If you don't want to install all the dependencies, which include geopandas and some other geospatial dependencies, you can use the regular 'pip install' command.
root@ubuntu:~$ pip3 install verticapy
You can set up a new connection.
import verticapy as vp
# Creating a new connection
vp.new_connection({"host": "10.211.55.14",
"port": "5433",
"database": "testdb",
"password": "XxX",
"user": "dbadmin"},
name = "MyVerticaConnection")
# Connecting to the Database
vp.connect("MyVerticaConnection")
Create a VerticaPy schema for native VerticaPy models (that is, models available in VerticaPy, but not Vertica itself):
vp.create_verticapy_schema()
After creating a connection and the verticapy schema, you can start importing your own files (click here to download the iris dataset).
iris = vp.read_csv("iris.csv")
iris
If you don't have data on hand, you can load some well-known datasets. We start by creating a vDataFrame using a dataset. In this example, we use the 'titanic' dataset.
from verticapy.datasets import load_titanic
vdf = load_titanic()
We can now explore our imported dataset through the vDataFrame.
vdf
You filter your data with a pandas-like syntax.
vdf[["name", "age"]][(vdf["age"] > 30) & (vdf["age"] < 60)]
With the exception of aggregations, everything is happening in the database and nothing is loaded in memory. You can view the generated SQL code using the set_option function.
vp.set_option("sql_on", True)
vdf.describe()
VerticaPy is smart enough to not recompute the same aggregation twice. Each virtual column has its own catalog which will be updated as the user modifies it.
vdf["age"].catalog
Draw the dataset Correlation Matrix.
vdf.corr(method = "spearman")
We can turn off the SQL code generation.
vp.set_option("sql_on", False)
Use statistical tests.
from verticapy.stats import jarque_bera
jarque_bera(vdf, "age")
Two lines code is enough to prepare your data and train/test your ML models.
# Modules
from verticapy.learn.model_selection import cross_validate
from verticapy.learn.ensemble import RandomForestClassifier
# Data Preparation
vdf["sex"].label_encode()["boat"].fillna(method = "0ifnull")["name"].str_extract(
' ([A-Za-z]+)\.').eval("family_size", expr = "parch + sibsp + 1").drop(
columns = ["cabin", "body", "ticket", "home.dest"])["fare"].fill_outliers().fillna()
# Model Evaluation
cross_validate(RandomForestClassifier("rf_titanic", max_leaf_nodes = 100, n_estimators = 30),
vdf,
["age", "family_size", "sex", "pclass", "fare", "boat"],
"survived",
cutoff = 0.35,
cv = 3)
VerticaPy lets you to bring the logic to the data with just a few lines of code.
Enjoy!